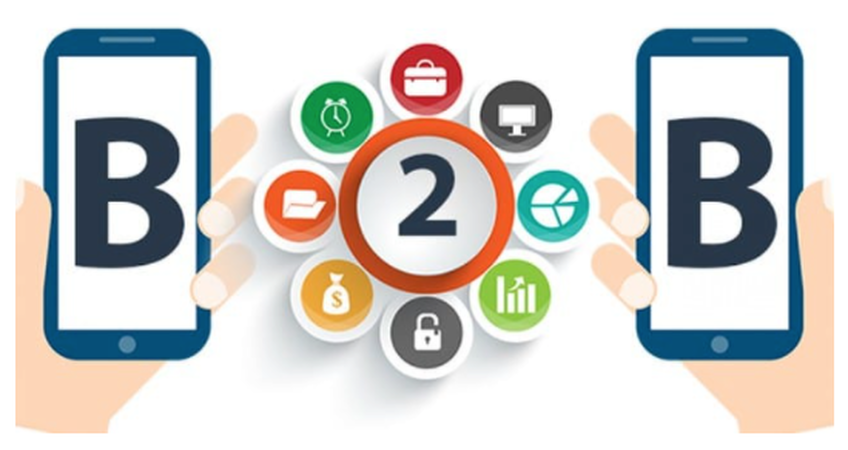
Can AI add value and scale to B2B business models? The short answer is a resounding “Yes”! One recent innovation has been how AI systems can automatically enrich product master data for large, complex product B2B databases.
Why is this exciting for B2Bs? The construction of rich product master data forms the foundation for B2B growth.
What is Product Master Data? And why is it important?
Product master data comprises all the information about a product necessary to present to a reseller network and/or sell products online.
For example, there are four standard groups of data sets:
#1. Product Identity Data:
- Long descriptive product name
- Product SKU
- Brand name (if different from Supplier)
#2. Standard Attributes:
- Product Description
- Technical specifications
- Sizing and colour information
#3. Merchandising Data:
- Feature image
- Complimentary image(s)
- Product video
#4. B2B Specific:
- Package sizes
- Packing/shipping characteristics (size and weight data)
- Delivery lead times
- Minimum order quantities (MOQs)
- Purchase volume discounts
- Kit configurations
The above generic list is a guide. Unique products require unique master data.
B2B Electronics Example:
Have a look at the laptop below from Best Buy USA, and consider the unique attributes needed for a laptop…
- Technical features (Bluetooth etc.…)
- RAM
- Laptop Model Family
- Operating system
- Processor Brand (Intel, Apple etc…)
- Inputs and Outputs
- Display (LED, LCD)
- Video Card
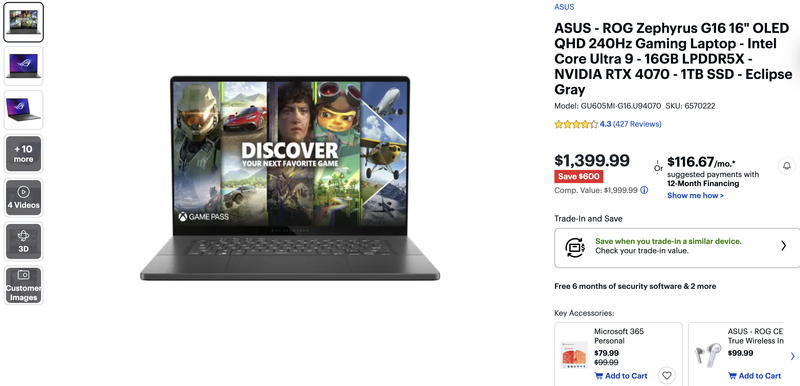
Best Buy expects this level of master data to come from suppliers.
Why is it crucial for B2B’s to own the Product Master Data Function?
The answer is simple yet often not taken seriously by B2B's: B2B organisations own the “Product Origin Story”.
What does this mean? A B2B organisation knows the following...
- Why was the product created?
- Why are specific attributes (a characteristic or feature) of a product valuable to a consumer market?
- How best to merchandise the product.
Whether a B2B organisation sells products to a retail network or has its own direct-to-consumer channel, it is the most qualified and suitable creator of product master data.
By translating the “product origin story” into product master data, a B2B organisation amplifies the retail network's ability to sell products. And this amplification is achieved at scale.
How and Why Does Master Data Drive Growth for B2B’s?
Rich product master data influences B2B growth in 3 different ways…
- Enriching digital B2B experiences for retail buying teams
- Drives new customer acquisition
- Supporting the retail network – when they win, you win
#1. Enriches B2B site experiences for retail buying teams:
Retail buying teams want to find and buy products digitally from B2B organisations. These buying teams demand a “consumer-like” digital experience. Why? Because they get these experiences in their personal lives.
Some recent research from Forrester (released October 2024)…
- 50% of B2B buying teams demand an Omnichannel experience.
- 94% of B2B CEO’s support and are leading digital transformations in their organisation.
- 74% of B2B leaders recognise they need to evolve their digital selling experiences.
Creating amazing “consumer-like” buying experiences on the B2B site depends on constructing rich and well-structured product master data. This enables a high standard of….
- Site search experiences
- Filtering experiences on product listing pages
- Product comparison experiences
#2. New Customer Acquisition – SEO and B2B Marketplaces:
Many B2BS are continuously searching for new customers to resell their products. A high standard of product master data is crucial for SEO and marketplace strategies.
While SEO is commonly known, the tactic of leveraging B2B Marketplaces is growing.
The number of marketplaces serving B2B organisations has grown from 75 in 2020 to over 600 today. The prediction is B2B marketplaces will grow to over 1,000 in the next 3 to 5 years!
Without a high standard of structured product data, any effort in leveraging a marketplace strategy will fail.
#3. Supporting Retail:
When a high standard of product master data is constructed, retailers are given data to amplify their own SEO function and Marketplace strategies.
Secondly, the retail business does not need to burn it's resources to feed their own business systems hungry for product master data (such as the eCommerce platform).
The retail network commonly does a poor job of filling the master data gaps because they are not the owner of the product origin story.
This from Amazon discussing the importance of product titles…
Your product title is one of the most important elements of your Amazon listing. It should be compelling, keyword-rich, and informative. Include primary and secondary keywords to improve search visibility.
Retailers need help and support with product master data and can't do this alone. There are three reasons as to why this is the case...
#1. A common retail strategy is to grow the product range:
A business can’t change its strategy because of a weakness in master data. The weakness needs to be changed to support the growth plan. If you provide strong master data you are supporting the retailer’s strategy.
#2. Retail product onboarding teams are lean:
Businesses are reluctant to throw headcount at issues, which is common (business) sense. The B2B supports a retailer’s ability to scale.
#3. Culture clash between buying teams and the retail business:
Some buying teams are used to buying for physical store networks and not online channels. As a result of this culture clash, product master data is not aggregated at the front end; retail onboarding teams are left scrambling.
But if the product master data is intact from the B2B, this becomes a non-issue.
AI - Scaling Product Master Data Enrichment:
An AI product enrichment system can support and scale the master data function by….
- Finding data gaps.
- Seeking out accurate information and populating data fields with the correct data.
- Taking existing data and reconfiguring it to support strategy (see product title example below).
- Audit product imagery and enhance images (see example below).
Where does AI find this information?
An AI system designed to enhance master data follows precise guardrails. These parameters define such things as...
- Where to look for the answers
- What language (wording) to use
- How to enhance images
- How to rearrange/modify existing data
Below are three examples of the types of guardrails that are working today to enrich product master data…
Example #1: Existing business libraries or siloed systems:
A business can have an existing siloed (stand-alone) system holding product data. The AI system can access this data and pull it into the master data in the correct format.
Think of a siloed business system holding schematic diagrams of spare parts.
Example #2: Business rules enhancing product titles:
The AI System can follow business rules to reconfigure existing master data. A great example of this is product titles. To develop a “product naming strategy” at scale, this type of naming process cannot be left to employees.
The laptop product title from Best Buy has been deliberately designed with attributes to support the strategic drivers mentioned above (SEO, Marketplace compliance, etc...).
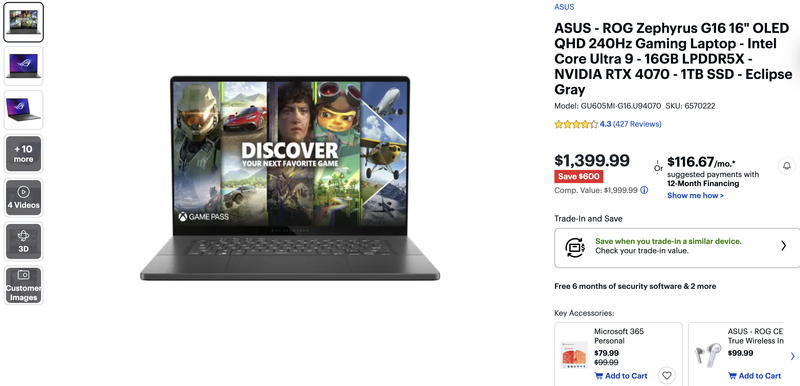
Example #3: Business Rules Enhancing Product Imagery:
AI can follow business rules to enhance product imagery. Image content can come in various forms…
- Dark or dirty backgrounds.
- No background at all when a background could assist in merchandising the product.
- Print quality (high resolution).
- Only showing a garment in a single colour when many colours are available.
- Incorrect dimensions for an eCommerce system.
- The image is named poorly (it is named after an obscure SKU—not ideal for SEO).
- Produce in-situ imagery (see training shoe example below).
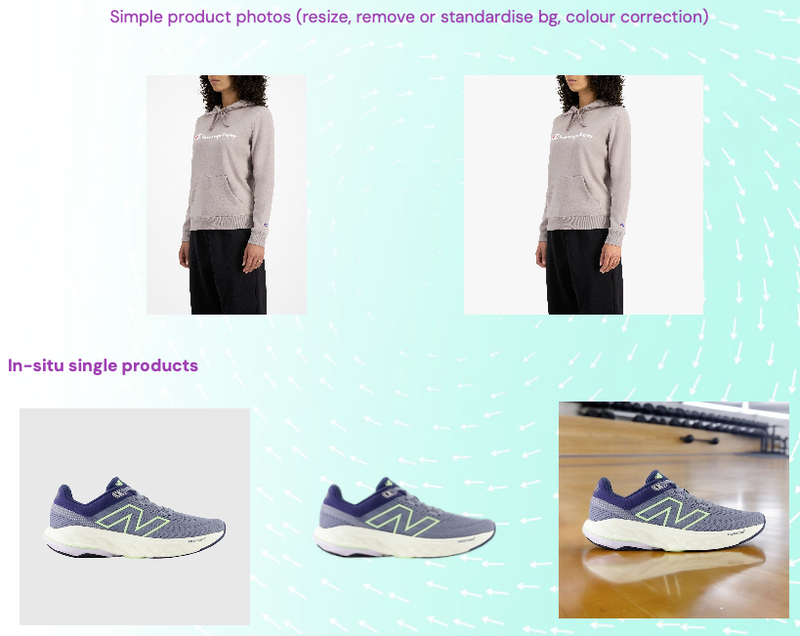
AI Enriching Product Master Data is Not Expensive:
Looking at the above, some may assume this is an expensive solution. It’s not!
To understand why, one must realise this AI system is a “Service as a Software”, not the typical “Software as a Service”.
“Service as a Software”:
AI solutions like the one above have flipped the script on the “Software as a Service” model, which refers to a business paying a license fee to access an entire software system.
Even though the business may only use 15% of the software, it pays for the whole system.
However, an AI-driven solution can be structured as a “Service as a Software”.
The “Service” is designed to support and scale a specific job or application a business needs, such as enhancing product master data. There is no need to pay for other features or functions the business does not need.
The “Software” is the infrastructure or the “engine” sitting behind the “service”, which the business does not need to bring in-house. This “software” is highly tuned to meet specific business needs.
Conclusion:
This method of leveraging AI sounds too good to be true. However, it’s a very real, powerful, and pragmatic way to produce tangible B2B value.
Nothing is sexy about it, and 99% of B2B businesses need it.
This article was as tagged as B2B , Best Practice , Ecommerce