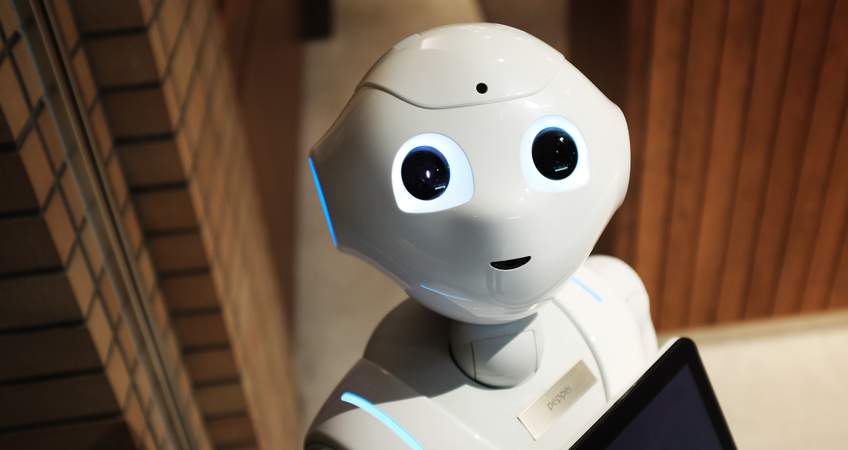
AI has produced an abundance of hype that has Retailers and B2Bs scrambling to find ways for it to add value.
A common use of AI is powering live chat systems to automate user interactions. While it broadens the capability to engage, this has yet to produce concrete wins simply because PEOPLE want to speak with PEOPLE when they need help or want a service. A great example of this can be seen with McDonalds.
McDonalds Example:
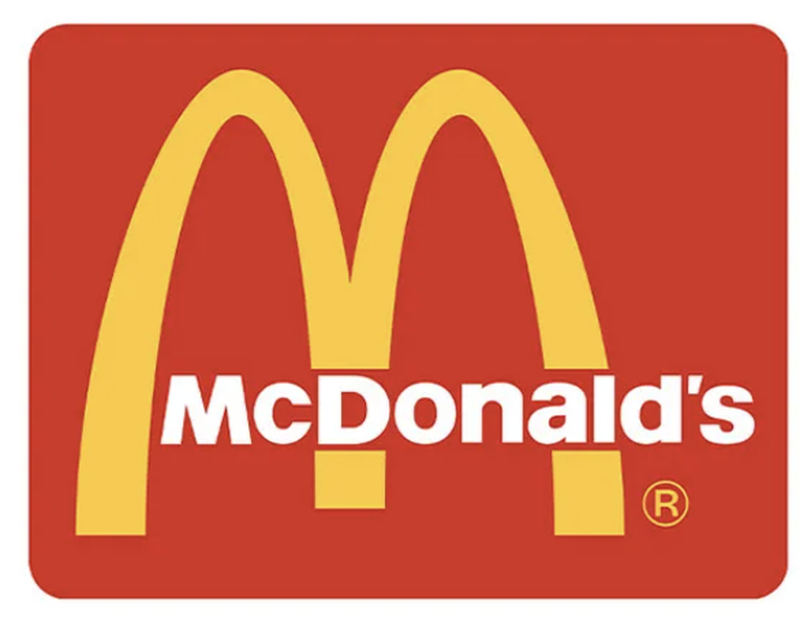
After three years of planning to use AI to take drive-thru orders, McDonalds USA, called the whole thing off in June 2024. The AI-driven chat repeatedly confused and frustrated customers.
Numerous social media videos showed how the AI-driven chat could not understand customer orders. In one video, the AI added 260 Chicken McNuggets to an order.
But don’t let these failures deter you.
One recent innovation has been how AI systems automatically enrich product master data.
Why is this exciting for retailers and B2Bs?
To answer this, a refresher on product master data and how it forms the foundation for growth is first required.
What is Product Master Data? And why is it important?
Product master data comprises all the information about a product necessary to present and sell it online. For example, in retail, there would be three standard groups of data sets:
#1. Product Identity Data:
- Long descriptive product name
- Product SKU
- Supplier name
- Brand name (if different from Supplier)
#2. Product Attributes Data:
- Product Description
- Technical specifications
- Product family or category classification
- Sizing and colour information
- Packing/shipping characteristics (size and weight data)
- Ingredients inclusions/exclusions (standard for beauty)
#3. Merchandising Data:
- Feature image
- Complimentary image
- Product video
- Product bundles (what can this product join to create a kit or other product)
- Product recommendations (what products complement this product)
B2B organisations would have data that relates to things like…
- Package sizes
- Delivery lead times
- Minimum order quantities (MOQs)
Laptop Example:
The above generic list of product master data is a guide. How a product meets and matches consumer needs will dictate the master data requirements for a product.
Take the laptop product seen below from Best Buy USA.
This retailer has successfully produced a master data list that includes such things as….
- Technical features (Bluetooth etc.…)
- RAM
- Laptop Model Family
- Operating system
- Processor Brand (Intel, Apple etc…)
- Inputs and Outputs
- Display (LED, LCD)
- Video Card
- The list goes on…
The list above is essential because these product attributes are important to consumers when buying a Laptop.
This is how and why product master data planning is strategic.
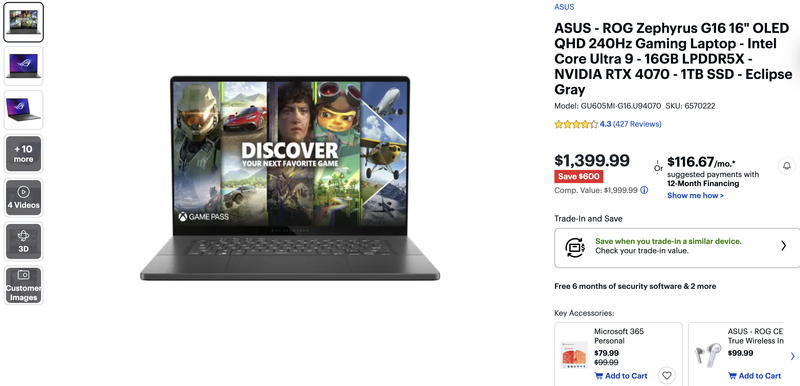
How Does Master Data Drive Growth? Why is it strategic?
After conducting a 5-year research project analysing the top-performing online retailers in the world (including Amazon), the one thing all top performers have in common is a high standard of product master data.
Product master data is competitive advantage.
A high standard of product master data amplifies…
- The SEO function
- Paid Search
- Paid Social
- Marketplace strategy
- Filtering within your website
- Site search experiences within your website
- Product comparison experiences within your website
Search engines, Marketplaces, and eCommerce platforms all need well-structured product data for these systems to promote, prioritise and present products.
This from Amazon discussing the importance of product titles…
Your product title is one of the most important elements of your Amazon listing. It should be compelling, keyword-rich, and informative. Include primary and secondary keywords to improve search visibility.
Sephora example:
One reason for Sephora’s digital dominance is its emphasis on product master data. People forget Sephora began as a “Pureplay” (online only).
The screenshot below is one section of a product page for a Shampoo product. All the information on the right-hand side is product master data in action. Notice how the “Ingredients” are listed on the site and not presented as a screenshot of the product label.
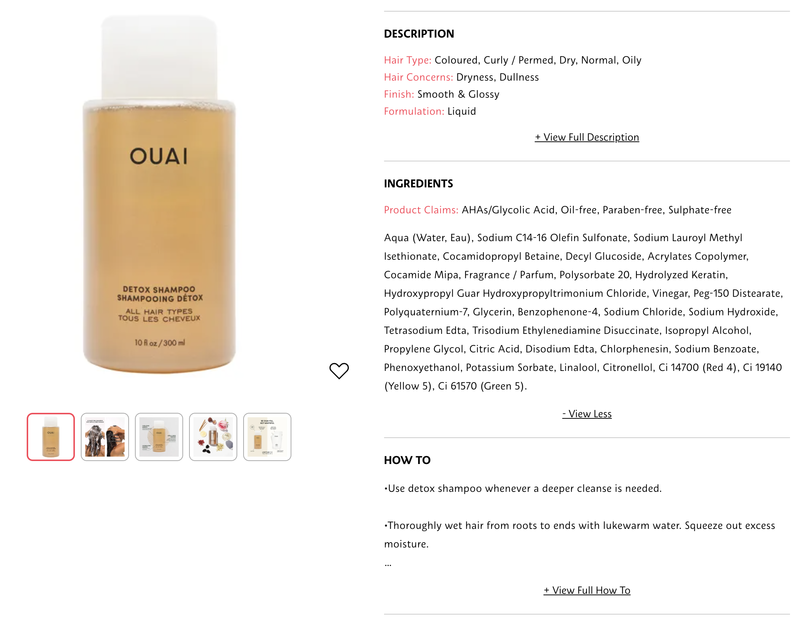
This is done intentionally so Google can index this master data. Sephora knows its customers are searching for specific shampoo ingredients and the exclusion of certain ingredients.
Under “Ingredients” notice how Sephora calls out “Paraben-free”! This is not an accident.
Product Master Data Pain Points - Not a simple fix:
Fixing product master data issues is not a simple fix for three reasons…
#1. A common strategy is to grow the product range:
A business can’t change its strategy because of a weakness in master data. The weakness needs to be changed to support the growth plan.
#2. Onboarding teams are lean:
Businesses are reluctant to throw head count at issues, which is common (business) sense.
#3. Culture clash between buying teams and the business:
Some buying teams are used to buying for physical store networks and not online channels. As a result of this culture clash, product master data is not aggregated at the front end; onboarding teams are left scrambling.
AI - Scaling Product Master Data Enrichment:
A product enrichment AI system can support and scale the master data function.
It can…
- Find and identify the data gaps.
- Seeks out accurate information and populate data fields with the correct data.
- Take existing data and reconfigure it to support strategy (see product title example below).
- Audit product imagery and enhance images (see example below). This includes the creation of swatches.
Where does AI find this information?
An AI system designed to enhance master data follows precise guardrails. These parameters define such things as...
- Where to look for the answers
- What language (wording) to use
- How to enhance images
- How to rearrange/modify existing data
Below are three examples of the types of guardrails that are working today to enrich product master data…
Example #1: Existing business libraries or siloed systems:
A business can have an existing siloed (stand-alone) system that holds product information. The AI system can access this data and pull it into the master data in the correct format.
Think of a siloed business system holding schematic diagrams of spare parts for B2B organisations.
Example #2: Business rules enhancing product titles:
The AI System can follow business rules to take existing master data and reconfigure it. A great example of this is product titles (mentioned above). To develop a “product naming strategy” at scale, this type of naming process cannot be left to employees.
The laptop product title from Best Buy has been deliberately designed with attributes to support the strategic drivers mentioned above (SEO, Marketplace compliance, etc...).
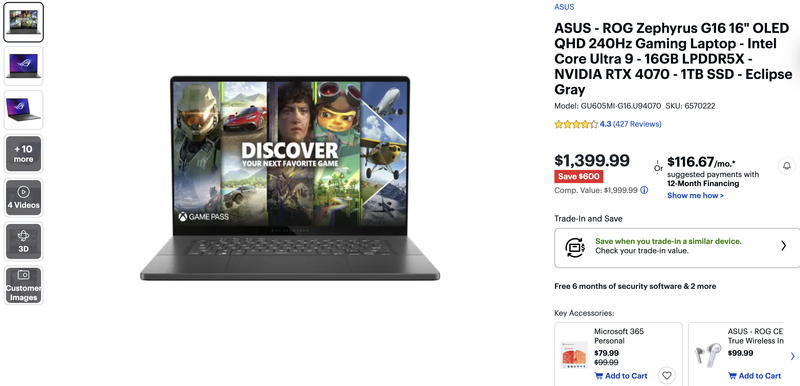
Example #3: Business Rules Enhancing Product Imagery:
AI can follow business rules to enhance product imagery. Many retailers use images that come from suppliers that suffer from…
- Dark or dirty backgrounds.
- No background at all when a background could assist in merchandising the product (see shoe example below)
- Print quality (high resolution).
- Only showing a garment in a single colour when many colours are available.
- Incorrect dimensions for an eCommerce system.
- The image is named poorly (the image is named as an obscure SKU – not ideal for SEO).
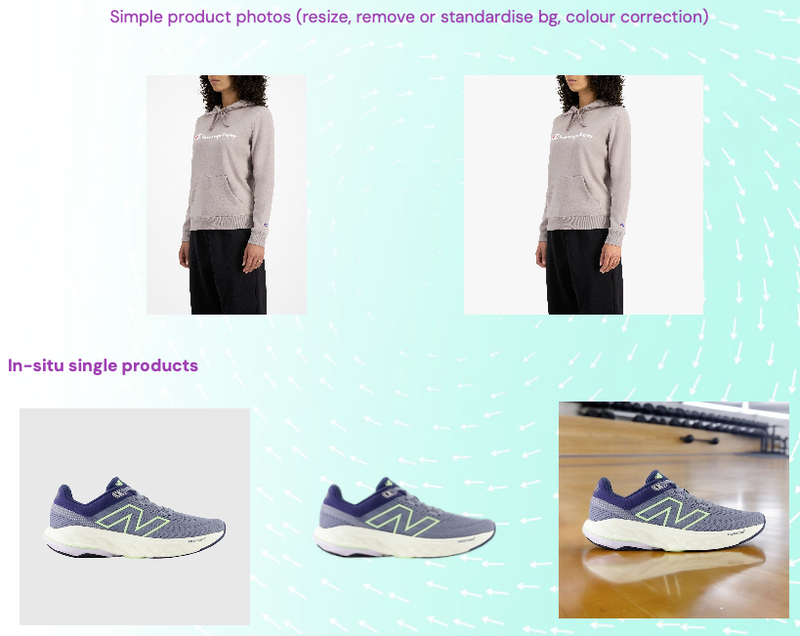
The business can dictate precisely how it wants images modified to accommodate systemic needs. Images can also be modified to support Google Shopping and Paid Social requirements, which differ from each other.
Google Shopping requires images in the following format…
- Non-apparel images - at least 100 x 100 pixels.
- Apparel images – at least 250 x 250 pixels.
- No image can be larger than 64 megapixels.
- No image can be larger than 16mb.
Meta's image format requirements...
- Product images are displayed in square (1:1) format.
- Images must be at least 500 x 500 pixels, but 1024 x 1024 pixels is recommended.
AI Enriching Product Master Data is Not Expensive:
Looking at the above, some may assume this is an expensive solution. It’s not!
To understand why, one must realise this AI system is a “Service as a Software”, not the typical “Software as a Service”.
“Service as a Software”:
AI solutions like the one above have flipped the script on the “Software as a Service” model, which refers to a business paying a license fee to access an entire software system. Even though the business may only use 15% of the software, it pays for the whole system.
However, an AI-driven solution can be structured as a “Service as a Software”.
The “Service” is designed to support and scale a specific job or application a business needs, such as enhancing product master data. There is no need to pay for other features or functions the business does not need.
The “Software” is the infrastructure or the “engine” sitting behind the “service”, which the business does not need to bring in-house. This “software” is highly tuned to meet specific business needs.
Conclusion:
This method of leveraging AI sounds too good to be true. However, it’s a very real, powerful, and pragmatic way to produce tangible business value.
Nothing is sexy about it, and 99% of businesses need it.
If you want to learn more about this solution, call or email Greg to learn more: Email: greg@commaconsulting.com.au Call 0477 723 474.
This article was as tagged as AI , Best Practice , Digital Strategy , Digital Strategy , Strategic Planning